Embedding Ethics and Governance in AI Solutions: A Practical Guide
- derekfmartin
- Feb 26
- 6 min read
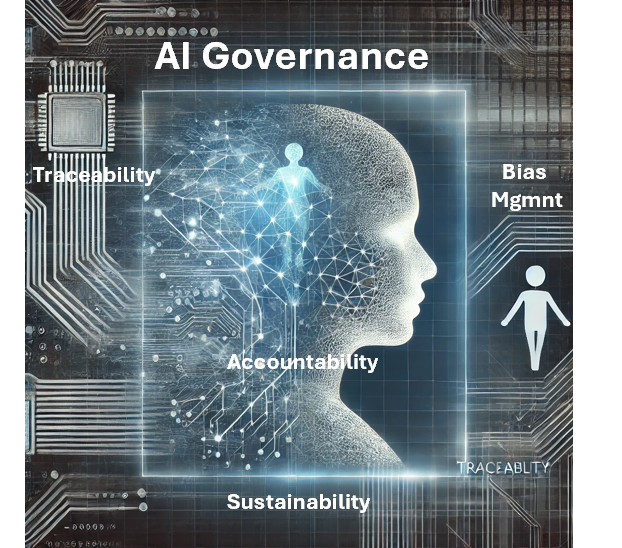
Article originally published on CMSWire.com.
The rise of artificial intelligence has reshaped industries, processes, and how we interact with technology. However, with great power comes great responsibility—embedding ethics and governance in AI isn’t just a "nice-to-have"; it’s a necessity. As organizations rush to adopt AI, ensuring these systems are ethical, transparent, and well-governed is critical to building trust and avoiding unintended consequences. Here’s how you can make ethics and governance central to your AI efforts.
1. Quantifying the Risks of Ethics and Governance Failures
Incorporating robust ethical standards and governance in AI solutions is not merely a moral imperative but a business necessity. Neglecting these aspects can lead to significant risks, including loss of trust, flawed analytics, and legal repercussions.
● Loss of Trust: Trust is foundational for any business. AI systems perceived as unethical or biased can erode customer confidence, leading to reputational damage. For example, Amazon's AI recruitment tool was found to favor male candidates due to biased training data. This led to the tool's discontinuation and tarnished Amazon's reputation. Reuters
● Flawed Analytics: AI systems lacking ethical oversight may produce biased or inaccurate outputs, resulting in flawed analytics that can misguide business decisions. The COMPAS algorithm, used in the U.S. criminal justice system to assess recidivism risk, was criticized for racial bias, inaccurately flagging Black defendants as high-risk more often than white defendants. Wikipedia
● Legal Risks: Unethical AI practices can lead to legal challenges, including lawsuits and regulatory penalties. Character.AI faced lawsuits alleging its chatbot encouraged harmful behavior among users. Business Insider
2. Establishing a Solid Foundation
Before diving into complex AI projects, pause and define your guiding principles. Ethics and governance need to be baked into your strategy—not bolted on after the fact.
● Define Ethical Standards: What values does your organization stand for? Ensure these principles are reflected in your AI objectives.
● Establish Governance Early: Create a governance framework that includes roles, responsibilities, and accountability measures for the entire AI lifecycle.
● Ensure Transparency: Avoid creating a "black box" solution with output that cannot be understood. Endeavor to build Explainability and Auditability into your solution.
Actionable Tip: Use version control systems and maintain clear documentation of all AI development steps.
2.1 Tackle Bias Early
Bias in AI isn’t just a technical problem—it’s an ethical one. Left unchecked, it can lead to erroneous and discriminatory outcomes that harm individuals and damage reputations.
● Diverse Data: Ensure your training datasets represent a wide range of demographics and scenarios.
● Continuous Auditing: Regularly test your models for bias and refine them based on findings.
● Cross-Functional Teams: Bring diverse voices into the AI development process, including ethicists, legal experts, and underrepresented groups.
Actionable Tip: Implement fairness-aware machine learning techniques to mitigate biases during model development.
2.2 Build Traceability into Your Systems
Traceability ensures you can track the entire lifecycle of an AI system—from data sourcing to decision-making.
● Data Provenance: Keep a detailed record of where your data comes from, how it’s processed, and where it’s used.
● Lifecycle Monitoring: Use tools to monitor AI performance over time, ensuring it continues to align with ethical and governance standards.
● Incident Reporting: Create a mechanism for reporting and addressing unintended consequences or ethical breaches.
Actionable Tip: Adopt tools like Model Cards or Data Sheets for datasets to document the details of your AI systems transparently.
3. Governance Isn’t a One-Time Task
AI governance isn’t a "set it and forget it" initiative—it’s an ongoing commitment.
● Dynamic Regulation: As AI evolves, so should your governance frameworks. Regularly review and update them to address new challenges.
● Stakeholder Engagement: Foster an environment where feedback from users and stakeholders informs governance decisions.
● Continuous Education: Equip your teams with the knowledge and tools they need to navigate the ethical and regulatory landscape.
Actionable Tip: Schedule periodic governance reviews to ensure your frameworks stay relevant and effective.
4. Empower Teams with Tools and Training
Ethical AI begins with the people building it. Equip your teams with the right mindset, skills, and resources to succeed.
● Ethical Training: Incorporate ethics into AI training programs for developers, data scientists, and business leaders.
● Low-Code Tools: Empower non-technical stakeholders to participate in AI projects using accessible tools that prioritize ethical considerations.
● Culture of Accountability: Foster a culture where ethical concerns are raised and addressed without fear of retaliation.
Actionable Tip: Use role-playing exercises to simulate ethical dilemmas your teams might encounter and develop their decision-making skills.
5. The Payoff: Trust and Sustainability
When organizations prioritize ethics and governance in their AI efforts, they don’t just avoid pitfalls—they build systems that are trusted, scalable, and resilient. By following these steps, you’ll not only comply with regulations but also create solutions that genuinely serve your customers and society.
The world of AI moves fast. Keeping ethics and governance at the heart of your strategy ensures that you’re not just moving quickly—but responsibly.
Ready to get started? Take one actionable step today: Review the frameworks and resource provided below to select the best approach for your needs. Use it to assess your current AI projects against these principles and identify gaps. Embedding ethics and governance isn’t just about doing the right thing—it’s about doing the smart thing.
Governance and Ethics Frameworks
1. OECD AI Principles
● Description: The OECD's AI Principles provide high-level guidance for governments and organizations on responsible AI development and use.
● Core Components:
○ Inclusive growth, sustainable development, and well-being.
○ Human-centered values and fairness.
○ Transparency and explainability.
○ Robustness, security, and safety.
○ Accountability.
● How to Use: Organizations can align their AI strategies with these principles, incorporating them into governance policies and decision-making frameworks.
● Source: OECD AI Principles
2. AI Fairness 360 Toolkit (IBM)
● Description: A comprehensive open-source toolkit developed by IBM to help developers detect and mitigate bias in machine learning models.
● Core Features:
○ Pre-built bias metrics and fairness algorithms.
○ Tutorials and examples for diverse use cases.
○ Tools for auditing datasets and models for fairness.
● How to Use: Integrate this toolkit into the AI lifecycle to assess fairness during model development and deployment.
● Source: AI Fairness 360 Toolkit
3. EU Ethics Guidelines for Trustworthy AI
● Description: The EU's High-Level Expert Group on AI released these guidelines to ensure AI systems are lawful, ethical, and robust.
● Core Components:
○ Ethical principles: respect for autonomy, prevention of harm, fairness, and explicability.
○ Seven requirements: accountability, data governance, diversity, non-discrimination, and more.
○ A practical self-assessment checklist for AI projects.
● How to Use: Apply the self-assessment checklist during the AI design and deployment phases to ensure compliance with ethical standards.
● Source:https://op.europa.eu/en/publication-detail/-/publication/d3988569-0434-11ea-8c1f-01aa75ed71a1
4. Model Cards for Model Reporting (Google)
● Description: A template for documenting machine learning models' intended use, limitations, and performance across different contexts.
● Core Features:
○ Information on datasets used for training and testing.
○ Details on model performance, fairness, and biases.
○ Transparency in intended use and limitations.
● How to Use: Include model cards as part of your AI governance documentation to enhance transparency and accountability.
● Source: Model Cards for Model Reporting
5. AI for Good Impact Initiative
● Description: Led by the International Telecommunication Union (ITU) under the UN system, the AI for Good Impact Initiative focuses on harnessing AI to achieve the United Nations Sustainable Development Goals (SDGs). It serves as a global platform for collaboration between businesses, governments, and academia to apply AI responsibly.
● Core Components:
○ Global Collaboration: Connects AI innovators with problem-owners from various industries to tackle societal challenges like climate change, healthcare, and education.
○ Ethical AI Deployment: Promotes the development of ethical AI solutions that align with human rights and international standards.
○ Sustainability Focus: Ensures AI applications are designed to address pressing global issues, from poverty alleviation to environmental conservation.
○ Capacity Building: Offers guidance, toolkits, and resources to help organizations implement AI solutions responsibly.
● How to Use:
○ Engage with the initiative’s Innovation Factory to discover, prototype, and scale AI solutions for real-world impact.
○ Leverage their guidelines and ethical frameworks to design AI systems that are equitable, sustainable, and transparent.
○ Participate in workshops and forums to collaborate on AI projects targeting specific SDGs.
● Source: AI for Good Impact Initiative
6. Smart Industry Readiness Index (SIRI)
● Description: Developed by the Singapore Economic Development Board, SIRI provides a structured framework to assess and improve digital and AI governance in manufacturing.
● Core Features:
○ Focuses on three pillars: process, technology, and organization.
○ Includes 16 dimensions for evaluating AI maturity and readiness.
○ Offers a formal assessment process and improvement roadmap.
● How to Use: Use SIRI to identify governance gaps, prioritize improvements, and implement best practices tailored to your organization's AI maturity.
● Source: Smart Industry Readiness Index (SIRI)
How to Incorporate These Frameworks
Assessment: Start with self-assessment tools like the EU's Trustworthy AI checklist or SIRI to evaluate your current practices.
Bias Mitigation: Use AI Fairness 360 or similar tools to ensure fairness in your datasets and models.
Documentation: Adopt Model Cards to enhance transparency and traceability.
Principle Alignment: Align your AI efforts with global standards like OECD or EU guidelines for robust governance.
Continuous Improvement: Revisit these frameworks periodically to adapt to evolving regulations and organizational needs.
Comments